In the past few months, the topic of biases and fairness in our society has become widely discussed in the media. More and more evidence started to emerge of various occurrences of discrimintation including biases in recommender systems, biases in predictive policing algorithms, and biases in risk assessment to mention just a few.
In parallel, the topic has attracted the statistical community with a number of papers (even books) published and conferences dedicated to it. While there has been an increasing interest in the statistical and computer science communities, businesses are also actively investigating this area.
JP Rangaswami comments:
Many firms use some form of predictive analytics, machine learning and even artificial intelligence in their day-to-day operations. For many, the use may be about optimisation and load balancing within the physical infrastructure and the network. Some may have ventured further and started to use such techniques in earnest in recommendation processes, both internal (eg vendor selection for commodity products, sales funnel “propensity to buy” analysis) or customer-facing (eg product or service recommendations). Some may have gone even further and explored use in pricing, in product design, perhaps even in hiring.
In each case, when it comes to designing and refining the predictive process, it is likely that some datasets are selected specifically in order to train and to test the technology. And that’s where risks can emerge caused by biases in data.
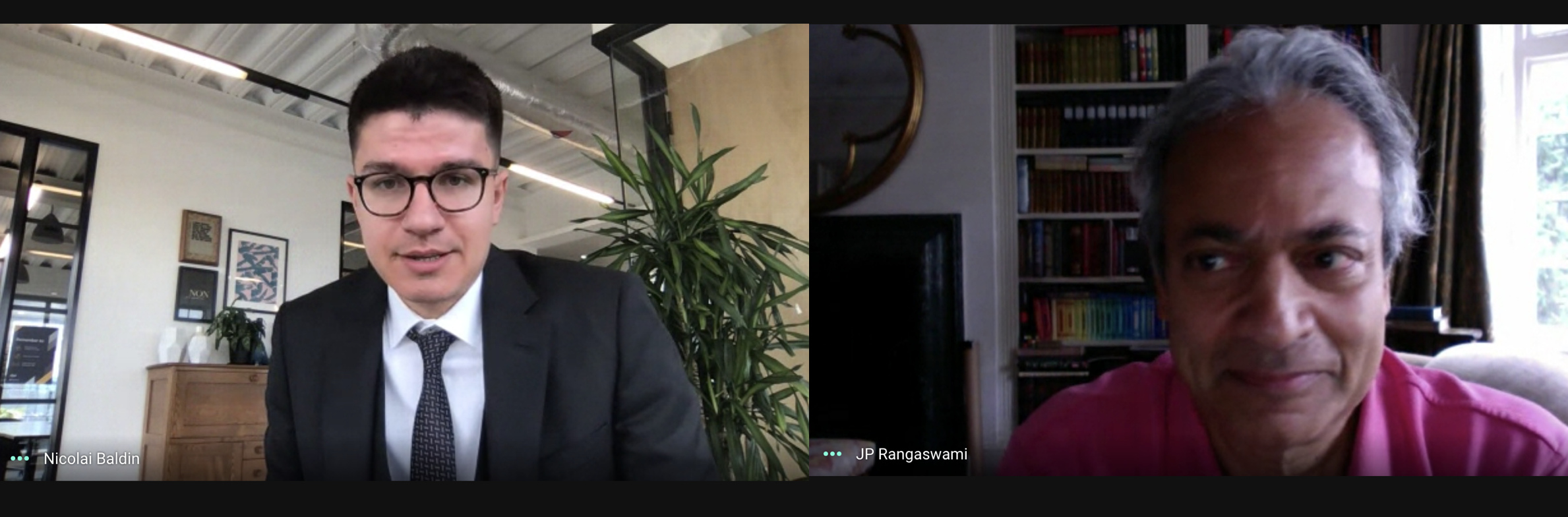
Nicolai continues:
Yet, the main confusion about this topic in the business community is driven by a common argument that statistical algorithms inherently rely on biases in data and therefore do discriminate. Is this really true? Unfortunately, there is no short answer to this question, but we aim to provide as much clarity in this post as we can to shed light on this complex issue of bias and fairness in machine learning.
Common misconceptions about biases and consequences.
Misconception 1: Removing sensitive attributes from data will make the dataset unbiased and fair.
Data and compliance officers who attempt to identify the presence or absence of bias in data typically observe an individual’s attribute (e.g. nationality) and a particular outcome (e.g., credit score) of a credit scoring model and try to determine whether that outcome would have been different had the individual had a different value of the attribute. In other words, to measure discrimination one must answer the counterfactual question: What would have happened to another individual if he or she had been Chinese? This is crucial for understanding fairness in machine learning.
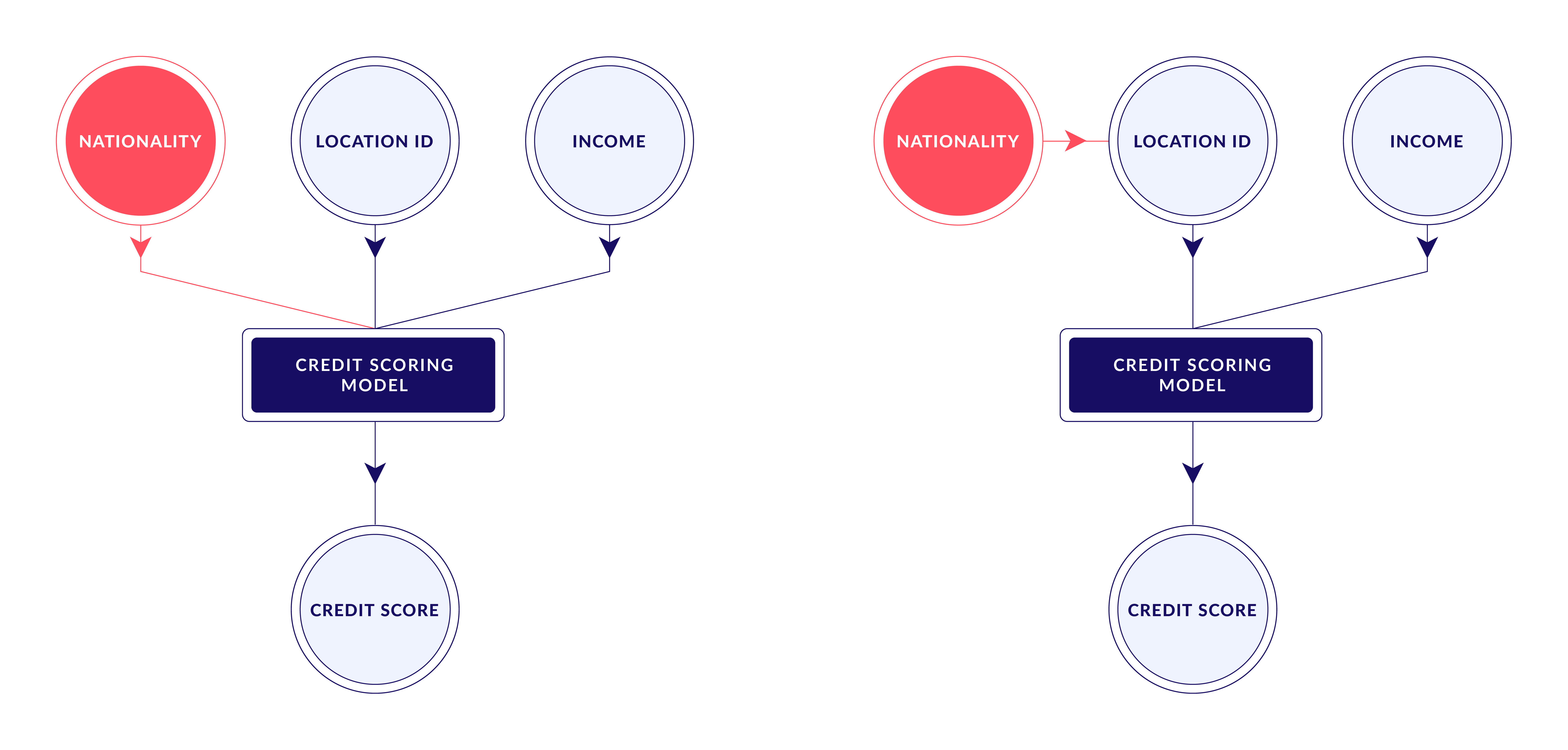
Answering this question is often not enough because of the causal effects and sufficient statistics hidden in data itself. In a nutshell, often there are at first “innocent” attributes in data such as “Location ID” which may uniquely define the value of a sensitive attribute with high probability and therefore the model will factor in the bias through that causal effect. Addressing bias and fairness in machine learning requires a deeper understanding of these hidden factors.
Misconception 2: Fairness in ML is an abstract topic and doesn’t have implications to my business. Discrimination is the very point of machine learning.
JP Rangaswami comments:
Where the bias manifests itself in poor predictive or assessment capability, the loss is in efficiency and in effectiveness. There is always a cost, but at least this can be contained.
When it comes to recommendation processes, internal-facing failures are similar; there are costs but they can be contained. Inaccurate customer recommendations may be manageable when it comes to books to read or films to watch; when it comes to selection of travel route with time sensitivity there can be a cost, but again it’s manageable. But as such techniques move to the world of healthcare, diagnosis and recommended treatment, the issue of bias in training data becomes much higher-risk and can have significant adverse consequences, not just for the company but more importantly for the patient. Bias and fairness in machine learning are critical in these high-stakes scenarios.
Bias within the structured data can also manifest itself in making incorrect decisions when it comes to vendor selection and to staff hiring, and, as mentioned before, in pricing. Each of these has consequences, particularly when it comes to regulated markets. Understanding fairness in machine learning is essential for mitigating these risks.
Nicolai Baldin continues:
Whilst we must acknowledge the difficulty of mapping the science behind algorithmic biases and the law as well as the extra complexity that country-specific legislation brings upon, regulators have already started applying impact analysis under current anti-discrimination laws. This indicates the growing importance of bias and fairness in machine learning in regulatory contexts.
In 2019, the US Consumer Financial Protection Bureau (CPFB) claimed jurisdiction over Upstart, a company that used alternative data and algorithms to assess lending decisions, with respect to compliance with the Equal Credit Opportunity Act. The CPFB found that the Upstart model approved more loans and lowered interest rates across all races and minority groups, thus creating no disparities that required further action under the fair lending laws.
The US Department of Housing and Urban Development brought a case against Facebook alleging that it violated the prohibition on housing discrimination because its ML algorithms selected the housing advertiser’s audience in a way that excluded certain minority groups.
The fast adoption of ML in banking, insurance and government sectors is likely to have an even more significant impact on people’s life chances in the coming years. Consequently, it is natural to expect a stricter legislation around biases in data and fairness of decisions made by vital ML models. As such, addressing bias and fairness in machine learning will become increasingly important.
Misconception 3: Fairness is a property of an ML model, hence we need to ensure customer-facing models are not discriminatory.
Nicolai Baldin argues:
If there is a trend in a dataset towards one of the groups of attributes or clusters, that means there is a bias in the dataset. If the dataset has bias then a machine learning algorithm will factor it in when making a prediction. This is the nature of algorithms. For example, assume there is a bias towards a minority group committing a crime in a given dataset, as a result of not enough people being analysed and taken into account. If there is only a minority group of people present in the area the crime will naturally be higher by that group, but that doesn’t mean the minority group is actually prone to committing crime in general. The model trained on that biased data won’t be applicable to any other group.
JP Rangaswami continues:
Bias in the structured data chosen can be caused by heterogeneity of coverage, comprehensiveness of data record, longitudinal aspects, or even selection and transfer process.
Key observation from these misconceptions is that bias is a property of an underlying dataset.
In assessing the bias, the following questions are hence critical:
- How was the dataset collected?
- What is the quality of this dataset?
- Has it been benchmarked against other larger samples of data, global trends and proportions?
In the second part, we will share recommendations for enterprise and discuss ideal scenarios.
Further references:
Legislation in the United States
- Race (Civil Rights Act of 1964);
- Color (Civil Rights Act of 1964);
- Sex (Equal Pay Act of 1963; Civil Rights Act of 1964);
- Religion (Civil Rights Act of 1964);
- National origin (Civil Rights Act of 1964);
- Citizenship (Immigration Reform and Control Act);
- Age (Age Discrimination in Employment Act of 1967);
- Pregnancy (Pregnancy Discrimination Act);
- Familial status (Civil Rights Act of 1968);
- Disability status (Rehabilitation Act of 1973; Americans with Disabilities Act of 1990);
- Veteran status (Vietnam Era Veterans' Readjustment Assistance Act of 1974; Uniformed Services Employment and Reemployment Rights Act);
- Genetic information (Genetic Information Nondiscrimination Act)
Legislation in the United Kingdom
Equality Act of 2010:
- age
- gender reassignment
- being married or in a civil partnership
- being pregnant or on maternity leave
- disability
- race including colour, nationality, ethnic or national origin
- religion or belief
- sex
- sexual orientation
References:
- 5 Types of bias & how to eliminate them in your machine learning project
- FairML : ToolBox for diagnosing bias in predictive modeling
- Local Interpretable Model-Agnostic Explanations (LIME): An Introduction
- Attacking discrimination with smarter machine learning
FAQs
What are some common sources of bias in machine learning datasets?
Common sources of bias in machine learning datasets include underrepresentation of certain groups, historical inequalities reflected in the data, and biased data collection methods. These sources can significantly impact bias and fairness in machine learning models.
How can businesses address bias and fairness in machine learning during the data collection phase?
Businesses can address bias and fairness in machine learning by ensuring diverse and representative data collection, continuously monitoring for biases, and incorporating feedback loops to refine data quality. This proactive approach helps in maintaining fairness in machine learning processes.
What role do regulatory frameworks play in ensuring fairness in machine learning?
Regulatory frameworks establish guidelines and standards for fair practices in machine learning. They mandate transparency, accountability, and non-discrimination, helping organizations align their machine learning models with principles of fairness and bias mitigation.
Can machine learning models be completely free of bias?
It is challenging to make machine learning models entirely free of bias due to inherent data imperfections and historical biases. However, through diligent efforts in data preprocessing, model training, and evaluation, businesses can significantly reduce bias and enhance fairness in machine learning.
What are the business implications of not addressing bias and fairness in machine learning?
Failing to address bias and fairness in machine learning can lead to legal consequences, reputational damage, and financial losses. Ensuring fairness in machine learning is crucial for maintaining customer trust, compliance with regulations, and achieving sustainable business growth.