For data to be useful, it needs to be shared, whether within a company between departments or with partners. Companies currently waste time, money and resources on suboptimal solutions to make it shareable in a privacy-compliant manner, or often do nothing with the data to avoid privacy and compliance issues. At Synthesized, we help partners solve this problem by using advanced machine learning and information security techniques: instead of sharing original data, we enable companies to work with compliant synthetic datasets mimicking the structure of the original data without disclosing any information about individual data points.
For the last two years, we’ve been developing our core product to address the problem for sensitive personal data and are currently helping our partners in product adoption.

What led you to create the business?
I have been working in machine learning and statistics for the last 8 years, and have collaborated with leading financial institutions in the US and the NHS in the UK. I was actually working on deep learning in early 2011 building recurrent neural networks with CPUs and Newton’s method before GPUs and stochastic gradient descent became the thing!
As I worked on some of the most advanced areas in ML, I became frustrated by the difficulty of obtaining quality test data due to compliance barriers and realised how massive the gap is between what the scientific community has developed and what it actually delivered to the world, mostly because of lack of infrastructure and communication with data. That’s how the idea for Synthesized was born around 2016.
Can you explain the underlying technology in more detail?
The underlying tech behind the main product is a high-dimensional machine learning engine that generates synthetic data sets by learning and reinforcing the structure of information found in original data. Based on recent advances in modern machine learning, our software is able to move beyond surface statistics to capture and reproduce the complex multi-dimensional patterns underlying realistic data sets. Unlike anonymization and encryption techniques, this approach enables our partners to share better quality information in a format useful for machine learning and analytics, without disclosing any information about individual data points.
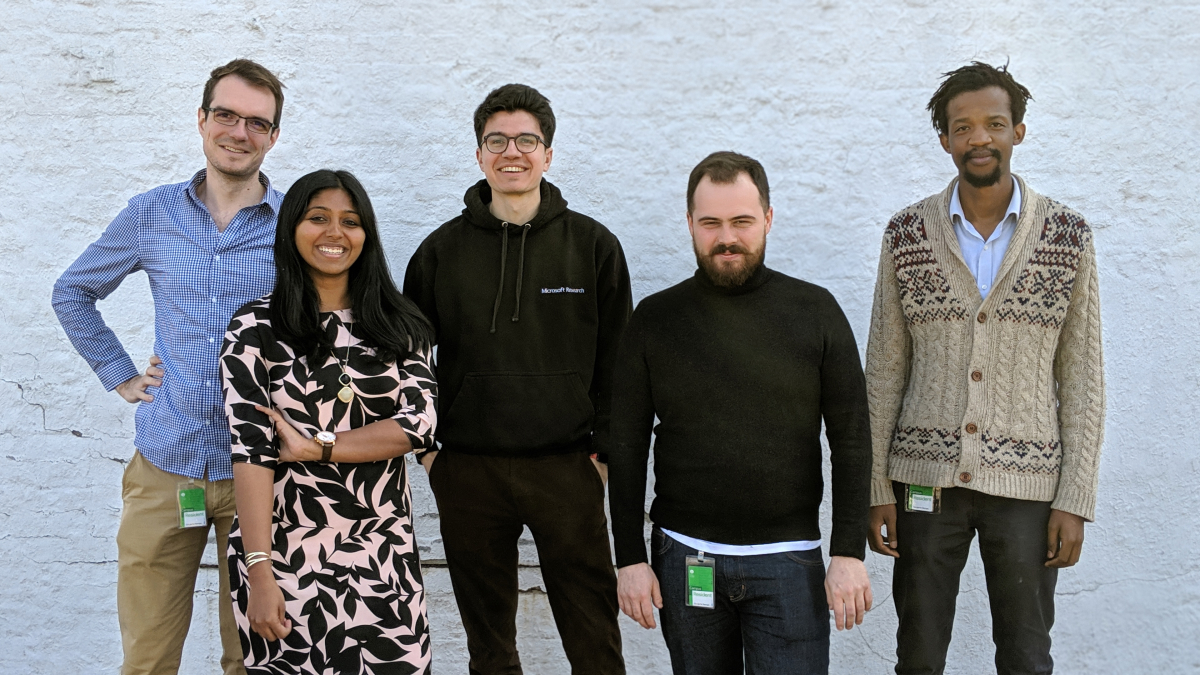
Who is in the team?
When working on an advanced machine learning product, talent is key. Our founding team alone combines over 30 years of first-hand experience working on machine learning products in leading enterprises and research institutions. The founding team members invested two years building the core platform for data synthesis upfront by developing proprietary algorithms and novel frameworks. Our business development is led by two commercial experts with over 40 years of combined experience in driving product adoption. We’re supported by a range of technology veterans including ex-CDO of Deutsche Bank, JP Rangaswami, ex-CTO of Saxo Bank, Sergey Vidyuk, Staff Engineer from Google, Vitor Rodrigues, and investors that include Seedcamp and active support from the tech giants Google and Facebook.
What have been some of the key milestones on the Synthesized journey so far?
Sourcing talent and hiring the right people to complement the founding team’s skill set was a real priority over the last six months and we are now happy we’ve managed to piece together the missing pieces. At the beginning of this year, we completed the beta version of the main product which has already been successfully tested by two Tier 1 financial institutions in the UK and a multinational consultancy company. The commercial team also managed to better tailor our commercial model to our partners’ needs and refine product positioning for them.
What is your vision for the future of data?
We want to help create a world where data is truly an asset for both enterprises and consumers that help generate the data. ‘Big data’ doesn’t need to be big and daunting. Knowledge and insights can be unlocked just as well from synthetic data without jeopardising the trust that people placed in companies.